AI’s Impact on the Healthcare Consumer: A Path Forward for the Health Payer
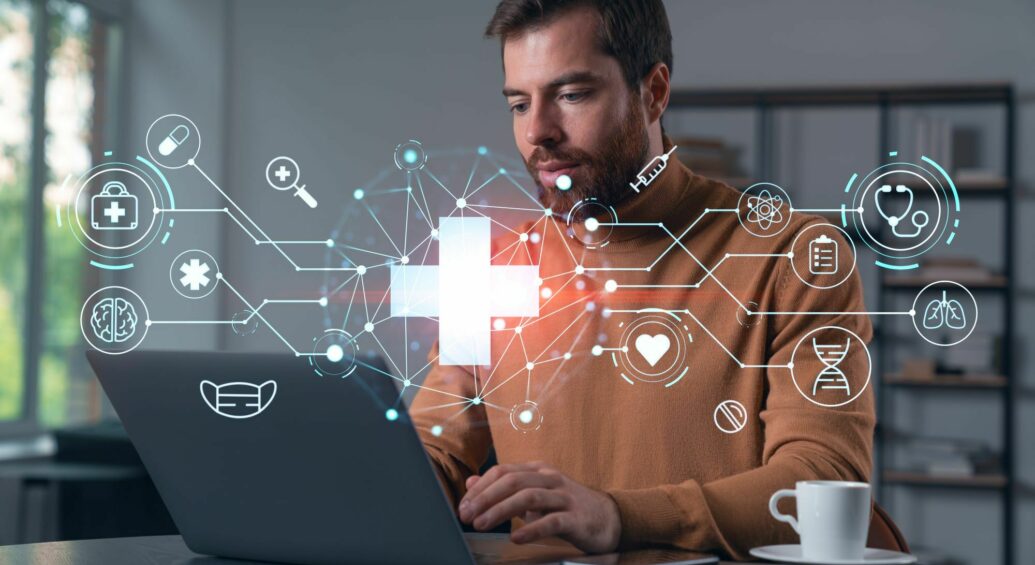
Individuals and businesses are embracing Artificial Intelligence (AI) technologies at an incredibly rapid pace. It’s been widely reported that ChatGPT attained a million users within 5 days – that’s 250 times faster than Netflix and about 15 times faster than the iPhone. While this comparison admittedly includes apples and oranges, it underscores the continued, and literally exponential, growth in the use of AI to transform businesses across industries over the next few years.
Various forms of AI have been in use for decades, and the healthcare industry has benefitted by addressing use cases like predictive models, sentiment analysis, chatbots, and more. What appears to be unique about the advent of Generative AI (GenAI) tools, such as ChatGPT, are the following:
- Vast Data Availability & Processing Power: The underpinning availability of large data sets and advanced processing power – these are enabling more rapid and diverse training of models.
- Advanced Algorithmic Approaches: The combination of Machine Learning (ML) and Natural Language Processing (NLP) and related new algorithmic approaches – these are increasing the speed of innovation.
- User-Friendly Applications: The ease of use of the interface to address business challenges – this is broadening the set of potential users and consequently, the number of use cases that can be conceived and addressed.
Healthcare is an industry with high potential for disruption by emerging AI capabilities. Some of the greatest areas of focus so far are:
- Patient Diagnostics: Automated interpretations of scans driving greater effectiveness and efficiency.
- Drug Discovery and Development: Using AI to analyze large data sets faster.
- Administrative Process Automation: Driving efficiency through transcribing voice to text, automating prior authorizations, and powering claims adjudication.
Yet, health plans, unlike Big Pharma and medical device industries, appear to be lagging in their efforts. Conversely, health plans may be on the brink of the biggest technology transformation opportunity of all time – development of breakthrough approaches to managing 1:1 relationships with their members. The healthcare consumer experience has been mismanaged by most health payers for many years, so there is a narrow window for some plans to seize a sustainable competitive advantage.
The Challenge
As a consulting firm solely focused in the healthcare space, we have been guiding our payer clients on their AI enablement journeys since 2019. However, we are concerned that health payers are:
- Not moving fast enough.
- Underestimating the significance of imminent industry disruptions.
- Failing to address core infrastructure barriers that hinder their ability to respond to rapidly changing market dynamics.
Currently, many health payers today are working toward AI governance and ethics policies, focusing on administrative cost reductions through the use of AI, and entrusting AI investment strategies to technical and strategy teams with limited understanding of the future of the healthcare consumer experience. While these are all critical areas to address, these efforts are slowing actual progress. New entrants, dramatic shifts in industry structure, and ever-increasing consumer expectations could jeopardize long-term business models, unless corrective action is taken.
A Path Forward
While health payers may be behind in the AI race, there is still time to remain competitive. And while there are many potential priorities for a plan to pursue, we recommend starting with the primary customer of the product – the healthcare consumer. The first order of business is to reimagine the relationship between the plan and the consumer in light of AI capabilities. Second, health payers must aggressively address their data problem. While AI can assist with analysis of both structured and unstructured data, consolidation of data and linking it to individual member records in a comprehensive and longitudinal fashion is imperative. Finally, get to work immediately – on something. On anything related to AI. While none of us can predict the future with certainty, initiating AI-related experiments at scale will accelerate organizational learning and inform both technology and human resource strategies.
Establish an AI-Enabled Vision of the Future
For decades, large enterprises have operated in a framework of choosing one or maybe two areas in which to excel to achieve competitive advantage – operational excellence, customer intimacy, or product leadership.3 Among most health payers it seems clear that customer intimacy has rarely been a primary, or perhaps even secondary, focus – despite the fact that payers may possess more consumer data directly attributable to its customers than any other industry. With the advent of emerging AI alongside selected other technological advances, health payers who move quickly have an opportunity to achieve competitive advantage by promoting customer intimacy as their primary competitive strategy. Even health payers who elect to remain focused on operational excellence will need to up their customer intimacy game as table stakes increase due to AI advances.
At this early stage of the transformation, different health payers may adopt different approaches to enhancing customer intimacy such that they remain differentiated from others who also do so. Elements of a new health payer member experience may benefit from any or potentially all of the following:
- Enhanced digital interfaces that yield improved relationships.
- More accessible individual health data collected from in-home devices.
- AI-driven next-best actions tailored to member needs.
- Cross-condition predictive models that provide holistic care insights.
- Real-time AI-driven reactions to nutrition triggers.
- AI-enhanced care coordination across multiple providers.
- Auto-diagnosis of conditions and diseases using advanced algorithms
- Lower-cost therapies personalized based on individual data.
- Even a redefinition of the health payer’s role in a member’s health.
The possibilities are vast – all of the above and many other line items are increasingly achievable due to more available data, more intelligent analysis, and more real-time reactions to that data and analyses using AI. Imagine what the health payer-to-member relationship would be when it’s based on an individual’s voluminous and well-understood data. For instance, a health payer might have the option of offering a newly defined plan custom-designed for that individual member based on data assessed in the middle of the plan year. That newly defined plan might more readily include aspects of care delivery not often contemplated – proactive data-driven diagnoses and disease remediation provided by health payer automation with no provider involved.
However, if a health payer has no vision for the future member experience, it will not be achieved.
Attend to the Member Data Problem
Member data within plans is typically spread broadly across departments and disparate technology platforms – portal data, eligibility data, claims data, intervention history data, engagement data, vendor data, and clinical / care management data. Additionally, structured data, unstructured data, and member messaging/content are managed in separate infrastructure for the most part. While AI technologies can assist in making sense of the various data across platforms over time, dirty data leads to poorly trained models.
Regardless of the health payer’s AI-enabled vision of the future, accelerating progress requires an immediate overall data cleanup project. Various off-the-shelf platforms are available to accelerate a health payer’s progress – Customer Data Platforms (CDPs) like Redpoint Global, Master Data Management (MDM) platforms like IBM Infosphere, and API platforms like Mulesoft from Salesforce can all play roles. Notably, many of these platforms have evolved to address multiple categories, and it is feasible to start with current third-party or home-grown platforms and augment them to fill gaps. However, taking on a large waterfall custom development project will be too slow. Again, the pace of innovation due to AI is too fast.
Additionally, developing the map of member data to platforms to a member intervention taxonomy is a critical path activity. An element of the consolidated member data set is member intervention history. Characterizing historical member interventions and then mapping them to individual member behaviors via a centralized and rationalized member record will inform emerging AI tools which in turn enables determination of optimized intervention approaches in the future state. Regardless of the particulars of an individual health payer’s AI-enabled vision of the future, understanding the relationship of interventions with their effectiveness at the individual member level will be table stakes.
Without clean data, AI is significantly less transformational.
Get Started Experimenting with AI Projects-- Now
AI has moved the healthcare industry to a place of unprecedented opportunity, but it also brings significant uncertainty. In past technology upheavals, remaining on the sidelines until the fog cleared was a legitimate strategy – the so-called “fast follower” strategy. Those days are over. AI technology innovation is moving too quickly, and those who wait may never catch up – by default the fast follower strategy may become the left-behind strategy.
Health payers certainly should manage risk as they proceed. Governance and compliance processes must be adapted to evaluate potential unintended consequences of AI investments and experiments. However, these processes can and should be more lenient with low-risk investments. There is clearly a difference between a human-in-the-loop approach for automated content generation and fully automating prior authorizations or allowing a chatbot to manage a claim denial. Few health payers have put in place overarching AI-enabled competitive strategies, but many have taken small steps. It’s critical for all health payers to at least get experiments underway. This will help the organization learn about new processes, understand the core technologies, and identify what are likely to be many human resource gaps.
Examples of low-risk AI projects that payers are undertaking to make incremental gains in an improved member relationship model include:
- Automated transcription and interaction summaries in Member Services.
- Automated coaching/prompting of outbound call center agents based on conversation analysis.
- AI-assisted knowledge management in Member Services.
- GenAI-driven optimization of outbound communications materials.
- Upgrading existing predictive models.
Health payers who do not act now may not have the opportunity to act in the future.
Conclusion
AI capabilities are improving at an ever-increasing rate, business models and processes are being redefined across industries, and health payers risk being left behind. Health payers that move decisively to leverage AI to redefine their relationships with their members have an opportunity to create a sustainable competitive advantage. These redefined relationships may be straightforward or transformational. And regardless of the degree of change and resulting differentiation envisioned, progress should be made immediately. Data must be rationalized, and experimentation must proceed at a minimum. In the past, moving too quickly was perceived as risky. In the new world of AI, moving slowly may be the riskiest strategy of all.
Contact us today to accelerate your organization's AI strategy and implementation.